In this article, we introduce a deep learning pipeline that extracts information from high-resolution aerial data. The solution extends our current machine learning processes that use an object-based approach for identifying tree seedlings using data captured by a cessna-mounted imaging system.
In this example, we take implementation one step further and use a deep learning segmentation model that extracts information from high-resolution aerial data. We test the solution over an area that includes recently planted pines and pockets of established and regenerating native bush.
The scaleable workflow developed uses data collected from the aforementioned airborne camera system and the results are a collaboration between Indufor and Scion’s Geomatics team.
Imagery used covers an area that was captured two-three months after planting at resolutions between 2.5 to 5 cm. The imagery collected was ingested into Indufor’s iterative deep learning framework designed to rapidly build high-quality datasets for deep learning.
A masked R-CNN neural network model pre-trained on the COCO dataset was trained on select areas of native forest and sprayed spots. The model builds on our pre-existing object detection model, used to identify spot spraying. Object detection models locate and represent objects with a bounding box, which suits features with relatively homogeneous size and shape. However, such a relatively crude method affects the model’s versatility, particularly when the targets are irregular and there is substantial variability between the geometry of targeted features (forests).
Comparatively, the two-class model combines the object detection algorithm with a semantic segmentation component which classifies each pixel in a bounding box. This enables sprayed spots and native patches to be accurately delineated (see the image below). The output can then be exported into common spatial formats for further analytics in a GIS and remote sensing environment. The detected sprayed spots are useful for forest managers to understand the quality of establishment. Automated mapping of the native vegetation is the first step in understanding and improving the biodiversity value of afforested sites. This is especially important as New Zealand looks to scale afforestation efforts to meet climate change targets.
We tested our model over a subset area of 16 ha. Within this area, we identified 13,295 discrete release spraying spots and 1,541 discrete blocks of native forest, both with an average confidence score of 80%. The confidence score dictates the confidence of the model in terms of the predicted feature. From these results, we also determined spot spraying occupied some 2.1 ha and native forest, 1.4 ha.
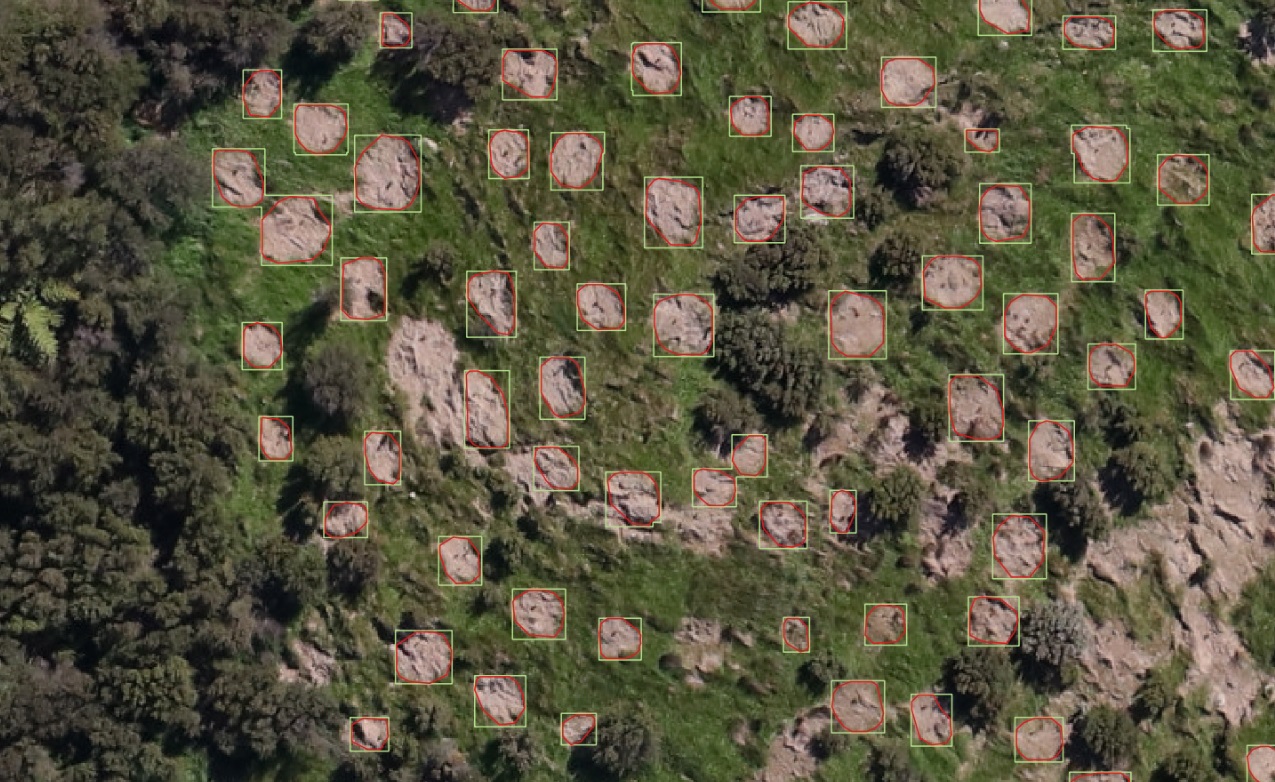
Comparison between object and segmentation-based outputs.
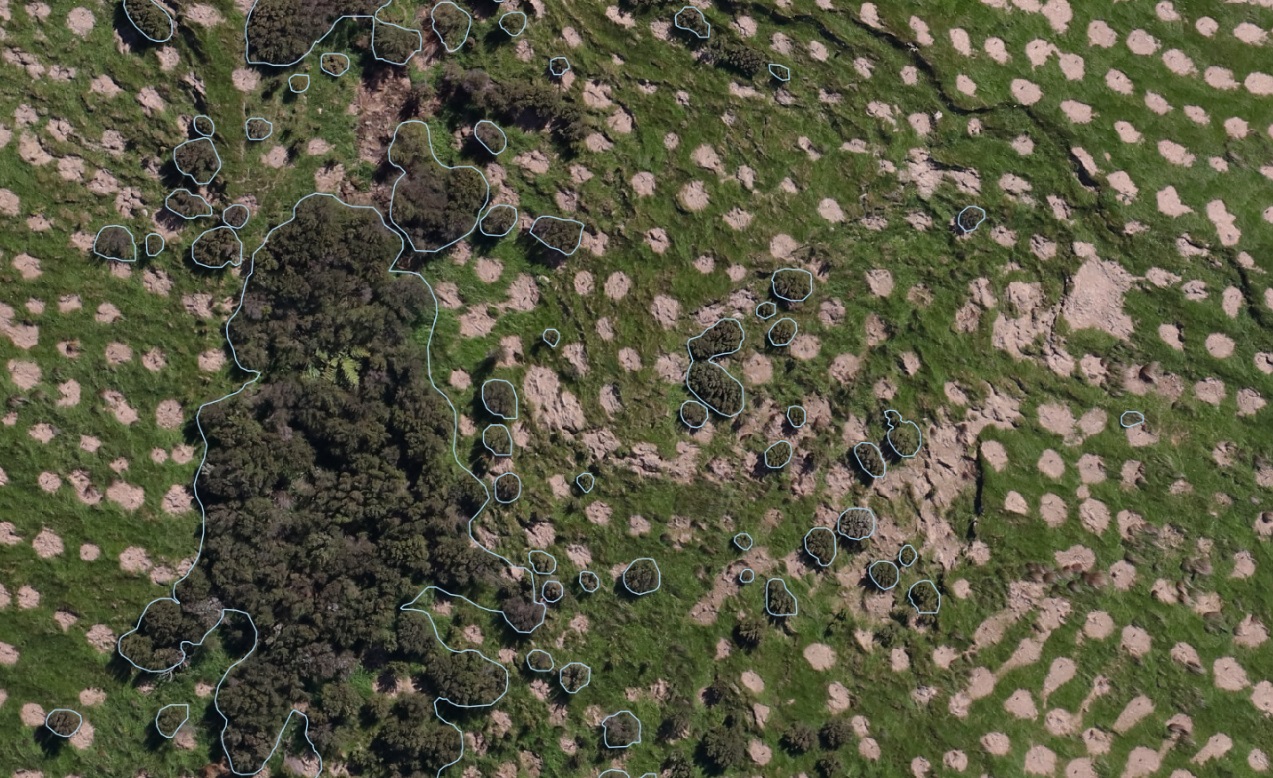
Native forest segmentation output.
Both object and segmentation-based approaches have unique applications which remain relevant within a spatial environment – where one excels over the other comes down to the use case. One key advantage of deep learning image segmentation is the ability to accurately delineate homogeneous landscape features. This provides spatial context and area metrics by class type(s). When integrating segmentation with cloud-based computing, it is possible to scale the monitoring area well beyond a localised (farm or woodlot) area. This introduces the potential for the segmentation approach to improve and refine national-scale databases such as New Zealand’s National Exotic Forest Description (NEFD) and LUCAS Land Use Map.
Indufor has invested heavily in deep learning capability over the past 18 months and we have seen excellent results across a range of tasks. We are excited to see what else is possible with these powerful new techniques.